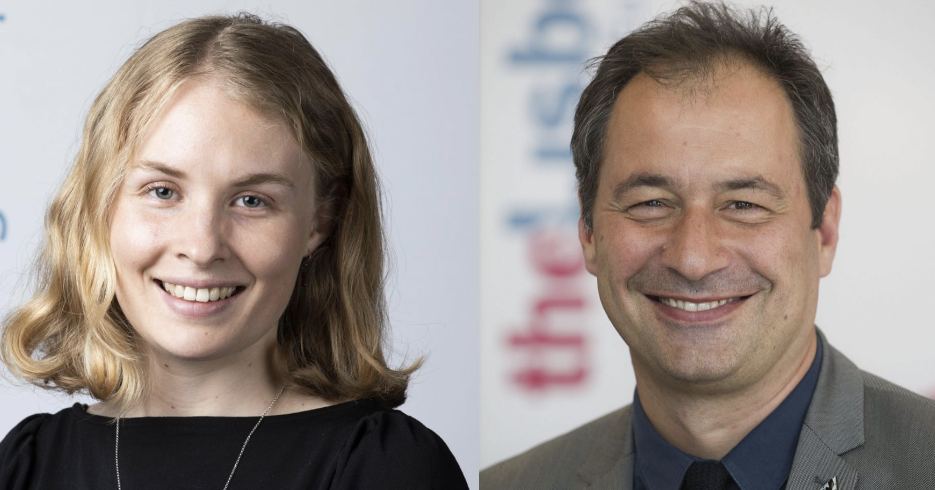
By Grace Milne, project manager and research associate, and David Osimo, director of research at the Lisbon Council.
On 08 December 2021, the Dutch data protection authority fined the tax administration for using for many years an algorithm that processed personal data beyond what was strictly necessary to identify people at risk of benefit fraud. Concretely, the algorithm identified high-risk families using, among other things, data on dual nationality, resulting in a de facto racist risk factor. As a result of this misuse, hundreds of children were removed from families and placed in foster care. The scandal was so large that the Dutch government resigned in January 2021.
This is a clear example of the kind of technology misuse that we are all worried about. And with good reason: the stories are horrific. Policymakers are prompt to react to this: The 2020 Berlin Declaration on Digital Society and Value-Based Digital Government calls for human-centric digital solutions that are “inclusive, help solve societal challenges and do not reproduce harmful social or economic biases.” The use of artificial intelligence in the public sector is the subject of policy proposals like the Artificial Intelligence Act, research and public debates.
The main problem in the Dutch case is not the algorithm or the misuse of personal data. It’s that, as it is often the case, data-driven innovation is used for fraud detection, rather than for improving social services. Over the last 30 years, the digitalisation of government has pervaded the welfare state in many layers of social security: eligibility and means assessments (Slovenia’s e-Sociala), fraud prevention and detection (Denmark’s welfare fraud analytics programme), and debt recovery (Australia’s online compliance intervention scheme). And meanwhile, around the world, technology is being used to enforce welfare controls like never before. Programs like Australia’s cashless debit card and the United Kingdom’s digital-by-default Universal Credit system monitor citizens’ adherence to welfare obligations to an unprecedented degree. To be clear, fraud detection is necessary and important: but at least a similar attention and investment should be devoted to improving the effectiveness of services. As the United Nations rapporteur on extreme poverty puts it, “instead of obsessing about fraud, cost savings, sanctions and market-driven definitions of efficiency, the starting point should be on how welfare budgets could be transformed through technology to ensure a higher standard of living for the vulnerable and disadvantaged.”
Regrettably, the Dutch algorithm failure is not the only example of data analytics in social services gone wrong. Australia's Online Compliance Intervention scheme (colloquially referred to as “robodebt”) was an automated debt recovery scheme active between 2016-2020 that incorrectly identified when welfare recipients owed money to the Commonwealth. It used a flawed algorithm that compared social security records with individuals' taxation data and resulted in wrongly-issued debt notices being provided to more than 400,000 people at the value of AU$1,73 billion (approximately €1,1 billion). Robodebt caused false debt notices to be issued to highly vulnerable people, often for large sums of money, who were then legally obliged to repay debts that they as welfare recipients had limited means of affording. In June 2021, the scheme was ultimately found to be illegal by the country's federal court and the Australian government settled the case for AU$1,8 billion (approximately €1,2 billion).
This focus on fraud detection and cost control is not driven by technology, but by long-standing policy trends. The first is the emergence of welfare conditionality: instead of people being entitled to welfare benefits on the basis of their citizenship, they are required to prove their eligibility through ongoing and often intrusive behavioural obligations (such as mandatory employment counselling or even drug testing). A second, even more important trend is the austerity objective that shaped government policies in the last twenty years, which made cost reduction the most important key performance indicator in digital government. One can agree or not with these policy objectives, but blaming inhuman outcomes on technology is incorrect and dangerous.
More precisely, the focus on the inherent dangers of technology such as machine learning has two negative consequences in the real world. First, by blaming technology, it removes responsibilities from decision-makers, thereby distracting from a much-needed and probably much harder debate about the new remit of the welfare state. Secondly, it empowers those who resist innovation and renewal in public services, in particular by limiting progress on data sharing, data analytics and the implementation of innovations such as the once-only principle. This unintended consequence has happened before: many officials report that the General Data Protection Regulation, despite providing many balanced options for allowing data processing, is often used as an excuse to avoid innovating government processes and share data across agencies, as we describe in a previous paper.
The human-centric alternative is not to limit the use of digital technology and revert back to analogue processes, which had plenty of biases too. It is to use technology to improve services and save lives, instead of punishing people. And this is not a far-fetched goal: it is already happening, and there are two emerging application areas for data-driven welfare services.
One is proactive service delivery: pre-registering people for services they have the right to. Research shows that complex registration processes affect disproportionately those in need, hence proactive service delivery will benefit them more directly. For instance, Portugal has implemented a proactive registration of citizens eligible for social energy tariff. By bringing together a wide range of data held by the government, the government automatically enrols citizens, without them having to request the aid. As a result of such proactive service delivery and automatic registration, 100% of the beneficiaries enjoy the benefits, which is 20 % of Portuguese families, at a time when energy costs are soaring and acting as a regressive tax. First embraced by Taiwan, proactive services are becoming more widespread among European cities, as shown in the UserCentriCities dashboard. One such service, the automatic registration of children to day-care by the city of Helsinki, just won the UserCentriCities award.
The second application area is data analytics for risk prevention: applying technology to more effectively identify people at risk, and intervene before the problem becomes unsolvable or tragedy happens. In other words, welfare services could benefit from the same radical improvements in terms of precision and predictive power, that have been seen in predictive maintenance for manufacturing and targeted watering for precision agriculture. One inspiring example is the UK Biobank, a database including genetic information on 500.000 citizens, which has been used by researchers to better understand the link between primary care and dementia, or the role of public health recommendations around diet and actual health outcomes. In Finland, the Health Benefit Analysis system screens data from 640.000 patients through 300 criteria to identify care gaps and prioritise high-risk patients. Proactive cancer screening, for instance, prevents 250 deaths per year through cervical cancer screening alone.
This kind of human-centric government is not only possible: it is required by the new historical context we find ourselves in. The pandemic, rising inequalities, the war in Ukraine and the related energy crisis have radically changed the perceptions on the role of government, where citizens have realised once again the importance of a strong safety net. Of course, one legitimate objection is that citizens mistrust government to handle their data well. But that is precisely because in the majority of cases data are used against people, such as to fight tax evasion, rather than to help them. And yes, data misuse is harmful and should be closely monitored, but missing the data opportunity also endangers and harms humans, in particular those in need. Data minimisation and bias avoidance should not be two absolute values, but part of a cost/benefit analysis. As Robert Kirkpatrick, director of the United Nation Global Pulse, puts it, the debate is too focussed on the misuse of data, rather than the missed use: “Lost opportunities to use big data to achieve the Sustainable Development Goals (SDGs) are probably to blame for at least as much harm as leaks and privacy breaches.”
Human-centric digital government is a historical shift, not just a declaration of intent. Digital government policies should be reoriented towards proactive and predictive services that help people. But how can this switch be achieved, for instance in the implementation of the Resilience and Recovery Fund? How do we deliver human-centric government while fully ensuring the data rights of the individual? What can we learn from the existing success stories? These are some of the questions that the UserCentriCities project is going to address over the next months. We’d love you to join us on this journey.
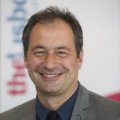